In recent years, there has been a significant rise in the use of Artificial Intelligence (AI) in healthcare. Among the different types of Data Science implementations, Generative AI has gained significant attention due to its potential to create new and unique data by generating realistic outputs. This has made it a valuable tool in various healthcare applications, including medical image analysis, drug discovery and development, personalized medicine, and disease diagnosis and prognosis.
Generative AI has immense potential in the healthcare industry. It can help in the development of new drugs, personalized treatment plans, and accurate diagnosis of diseases. It can also enable medical professionals to interpret complex medical images and extract valuable insights from electronic health records. The ability to generate new data using generative AI can help overcome the limitations of traditional data analysis techniques, which often rely on a limited amount of data.
In this blog post, we will explore the concept of Generative AI in healthcare and its potential applications. We will discuss the different types of Generative AI models, including Variational Autoencoder (VAE), Generative Adversarial Networks (GANs), and Autoregressive Models, and their respective applications. We will also examine the benefits and challenges of implementing Generative AI in healthcare, as well as best practices for successful implementation.
What is Generative AI?
Generative AI is a subset of machine learning that is designed to generate new data samples that are similar to the training data. The aim of Generative AI is to generate new data that is similar in structure, distribution, and other characteristics of the input data, thereby creating new data samples that can be used for a variety of applications.
Generative AI models work by learning from patterns in the input data and using this knowledge to generate new data samples that are similar to the input data. This makes it possible to generate new data that can be used for a wide range of applications in healthcare.
Large Language Models (LLMs) are a good example of Generative AI based on Natural Language Processing (NLP) algorithms.
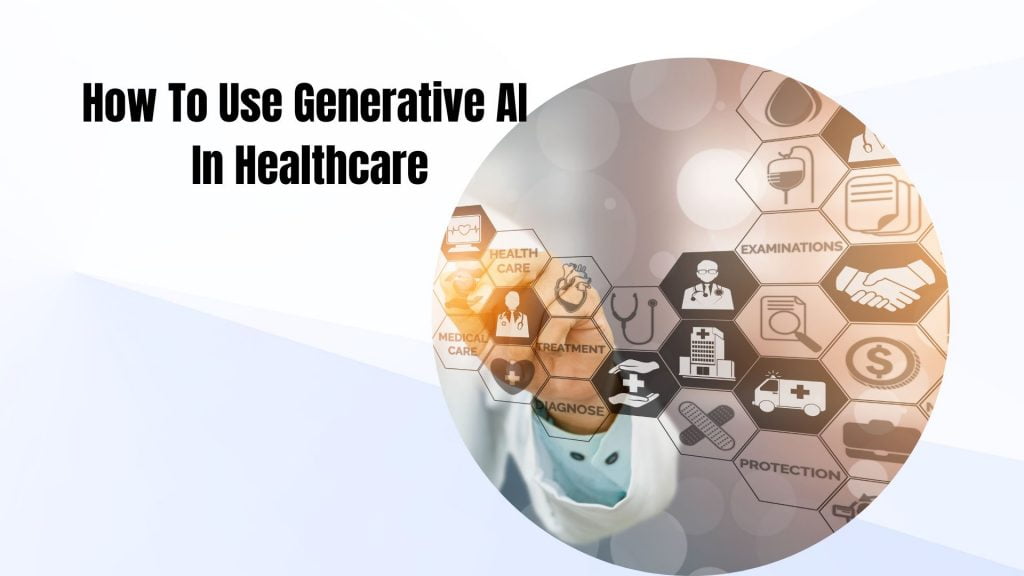
Use Cases of Generative AI in Healthcare
Generative AI is increasingly being utilized in the healthcare domain to solve complex problems and make better decisions. Here are some use cases of Generative AI in healthcare:
Medical Image Analysis
Medical image analysis is a crucial aspect of healthcare. The use of Generative AI models such as Variational Autoencoder (VAE) and Generative Adversarial Networks (GANs) has enabled medical professionals to obtain more accurate and detailed images of the human anatomy. VAEs and GANs can be used to generate high-quality medical images, enhance image quality, and detect anomalies
Drug Discovery and Development
Drug discovery and development is a time-consuming and expensive process. Generative AI models such as GANs and autoregressive models have been used to speed up the drug discovery process by generating new molecules and predicting their potential efficacy. These models have also been used to identify potential side effects of drugs and optimize drug dosages.
Electronic Health Record (EHR) Analysis
Electronic Health Records (EHRs) contain a vast amount of patient data that can be analyzed to improve patient outcomes. Generative AI models such as VAEs and GANs can be used to generate synthetic patient data that can be used for research purposes without compromising patient privacy. These models can also be used to identify potential risk factors for specific diseases and predict disease progression.
Personalized Medicine
Personalized medicine aims to tailor treatments to individual patients based on their unique genetic makeup, lifestyle, and medical history. Generative AI models can be used to analyze large amounts of patient data to identify patterns and predict how patients will respond to specific treatments. These models can also be used to generate personalized treatment plans for patients.
Disease Diagnosis and Prognosis
Generative AI models can be used to analyze medical images, patient data, and other sources of information to diagnose diseases and predict disease progression. For example, GANs have been used to identify cancerous tumors in medical images, while autoregressive models have been used to predict the progression of Alzheimer’s disease.
Generative AI is a powerful tool in the healthcare domain, and its applications are only just beginning to be explored. From medical image analysis to personalized medicine, the potential benefits of using Generative AI in healthcare are immense. However, there are also challenges that need to be addressed, such as data quality and regulatory compliance. By collaborating with domain experts and implementing best practices, the healthcare industry can leverage the power of Generative AI to improve patient outcomes and advance medical research.
Types of Generative AI Models
There are several types of Generative AI models that can be used in healthcare. Some of the most commonly used models include:
Variational Autoencoder (VAE)
VAE is a type of generative model that uses deep learning to generate new data samples. It works by learning a latent representation of the input data, which can then be used to generate new data samples that are similar to the input data. VAEs are commonly used in medical image analysis, drug discovery, and personalized medicine.
Generative Adversarial Networks (GANs)
GANs are another type of generative model that works by learning the distribution of the input data and generating new data samples that are similar to the input data. GANs work by training two neural networks – a generator network that generates new data samples and a discriminator network that distinguishes between the generated data and the real data. GANs are commonly used in medical image analysis and drug discovery.
Autoregressive Models
Autoregressive models are a type of generative model that works by predicting the next value in a sequence based on the previous values. They work by learning the conditional probability distribution of the input data and generating new data samples by sampling from this distribution. Autoregressive models are commonly used in electronic health record analysis, disease diagnosis, and personalized medicine.
Benefits of Using Generative AI in Healthcare
Generative AI has a significant impact on the healthcare industry, offering several benefits that can improve patient outcomes, enhance diagnosis accuracy, and reduce healthcare costs. Below are the key benefits of using generative AI in healthcare:
Speed and Efficiency
Generative AI models can analyze a vast amount of data in a short time and produce results with great efficiency. This speed and efficiency can be particularly useful in medical imaging, where large datasets need to be analyzed quickly to detect and diagnose conditions accurately.
Accuracy
Generative AI models can be trained to analyze medical data and generate accurate predictions with a high degree of precision. Medical image analysis using generative AI can identify and classify diseases with greater accuracy than human experts, leading to more precise diagnoses and improved patient outcomes.
Cost-Effective
Generative AI can help reduce healthcare costs by improving the efficiency of healthcare delivery. For instance, by automating routine tasks like data entry, clinicians can focus on patient care, thereby improving patient satisfaction and outcomes.
Reduction of Human Error
Human errors can occur in any medical process, and these errors can result in serious consequences, including misdiagnosis or delayed treatment. By leveraging generative AI, healthcare providers can reduce the chances of human errors, leading to better outcomes for patients. Generative AI can automate many processes, including medical image analysis and electronic health record (EHR) analysis, minimizing the possibility of errors caused by human oversight.
The benefits of using generative AI in healthcare are substantial. They include improved speed and efficiency, accuracy, cost-effectiveness, and reduction of human error. As such, it is not surprising that the healthcare industry is rapidly adopting generative AI to improve patient outcomes and optimize healthcare delivery.
Challenges in Implementing Generative AI in Healthcare
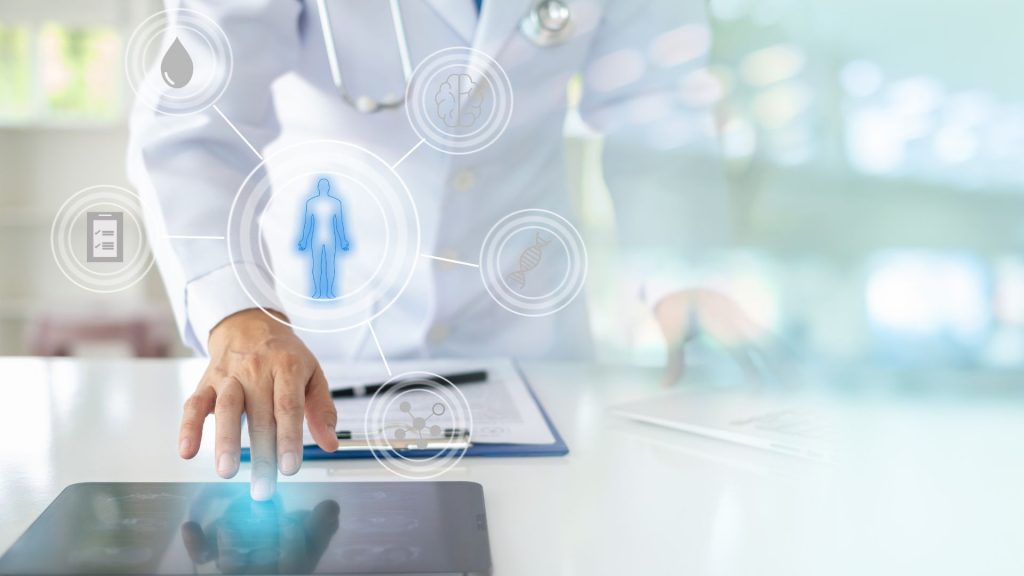
While generative AI has the potential to revolutionize healthcare, there are several challenges to its implementation in the healthcare domain. Some of the significant challenges include:
Data Quality and Quantity:
Generative AI models require vast amounts of high-quality data to learn and generate accurate outputs. However, healthcare data is often limited in quantity and quality due to several reasons such as data privacy concerns, data silos, and incomplete or inconsistent data. Generating synthetic data using generative AI techniques can help overcome this challenge, but the generated data must be validated to ensure its accuracy and authenticity.
Although there are some challenges in big data, it can serve as a valuable resource for training generative AI models, providing the large and diverse datasets necessary for generating high-quality content. The combination of big data and generative AI has the potential to drive innovation, creativity, and advancement in various fields.
Regulatory Compliance:
The healthcare industry is highly regulated, and there are several regulations and standards that must be adhered to, such as the General Data Protection Regulation (GDPR) and the Health Insurance Portability and Accountability Act (HIPAA). Implementing generative AI in healthcare requires compliance with these regulations, which can be a significant challenge, especially when dealing with patient data.
Ethical Considerations:
The use of generative AI in healthcare raises several ethical considerations, such as data privacy and patient confidentiality, potential biases in the generated outputs, and the accountability of the AI-generated recommendations or decisions. The ethical considerations must be carefully addressed to ensure that generative AI is implemented in a fair and just manner, and its benefits are distributed equitably.
To overcome these challenges, healthcare organizations must work closely with experts in AI, healthcare, and data privacy and security to develop appropriate strategies and frameworks. Moreover, implementing best practices and regulations can help ensure that the use of generative AI in healthcare is safe, effective, and ethical.
Implementing Generative AI Best Practices
Implementing generative AI in healthcare requires careful consideration and planning to ensure success. Here are some best practices to keep in mind:
Collaborate with Domain Experts:
Healthcare professionals and experts have valuable knowledge that can enhance the effectiveness of generative AI. By working together with domain experts, you can better understand the needs and limitations of healthcare, which can help in developing more effective generative AI models.
Ensure Data Privacy and Security:
Patient data is highly sensitive and needs to be protected. Any data used in generative AI models must be de-identified and kept secure to ensure patient privacy. This means implementing measures such as encryption, access controls, and data masking.
Conduct Extensive Testing and Validation
Before implementing generative AI models in healthcare, it is crucial to conduct extensive testing and validation to ensure the accuracy and reliability of the results. This involves testing the model on a large and diverse set of data to evaluate its performance, identifying and addressing any biases or errors that may be present, and verifying that the output generated by the model is clinically relevant and useful for healthcare professionals.
Testing and validation should involve collaboration with domain experts and healthcare professionals to ensure that the model’s output aligns with clinical expertise and knowledge. It’s also important to document the testing and validation process and results to demonstrate the model’s performance and address any concerns or questions that may arise.
Implementing generative AI models in healthcare requires careful consideration of the potential benefits and challenges, as well as adherence to best practices to ensure their accuracy, reliability, and ethical use. By collaborating with domain experts, ensuring data privacy and security, and conducting extensive testing and validation, healthcare organizations can successfully leverage generative AI to improve patient outcomes and advance medical research.
Future of Generative AI in Healthcare
Generative AI is an emerging technology that is constantly evolving and improving. With the advancements in deep learning and neural networks, generative AI models are becoming more sophisticated and capable of producing higher quality outputs. Researchers are also exploring new approaches to generative modeling, such as reinforcement learning and neuroevolution, which have the potential to further enhance the capabilities of generative AI.
The potential impact of generative AI in healthcare is enormous. By enabling faster and more accurate analysis of medical data, generative AI can help clinicians make more informed decisions about patient care. Additionally, generative AI can assist in drug discovery and development by simulating drug interactions and predicting the efficacy of potential treatments. This has the potential to revolutionize the pharmaceutical industry and lead to the development of new and more effective drugs.
As with any emerging technology, there are also ethical and regulatory implications to consider. Generative AI raises important questions about data privacy and security, as well as the potential for bias and discrimination in the algorithms. Additionally, there are concerns about the potential for generative AI to replace human workers in healthcare, which could have significant economic and social implications.
To address these concerns, it is important for healthcare organizations and regulators to establish clear guidelines and regulations around the use of generative AI in healthcare. This includes ensuring that data privacy and security protocols are in place, and that the algorithms are transparent and unbiased. It is also important to continue monitoring the impact of Generative AI on the healthcare industry and society as a whole, to ensure that its benefits are realized while minimizing potential risks.
Conclusion
In this blog post, we have explored the potential of generative AI in the healthcare domain. We have learned about the different types of generative AI models, their use cases, benefits, challenges, and best practices for implementing them in the healthcare industry.
Generative AI has already shown significant progress in various healthcare applications such as medical image analysis, drug discovery, and personalized medicine. It has the potential to revolutionize the healthcare industry by providing accurate and timely diagnosis, improving treatment outcomes, and reducing healthcare costs.
However, there are still challenges to overcome, such as data quality, regulatory compliance, and ethical considerations. To mitigate these challenges, it is essential to collaborate with domain experts, ensure data privacy and security, and conduct extensive testing and validation.
As Generative AI continues to advance, it will undoubtedly have a significant impact on the healthcare industry. With careful consideration of the ethical and regulatory implications, we can harness the power of Generative AI to improve patient care and enhance healthcare outcomes.